Nice Tips About When To Use Arima Three Line Break
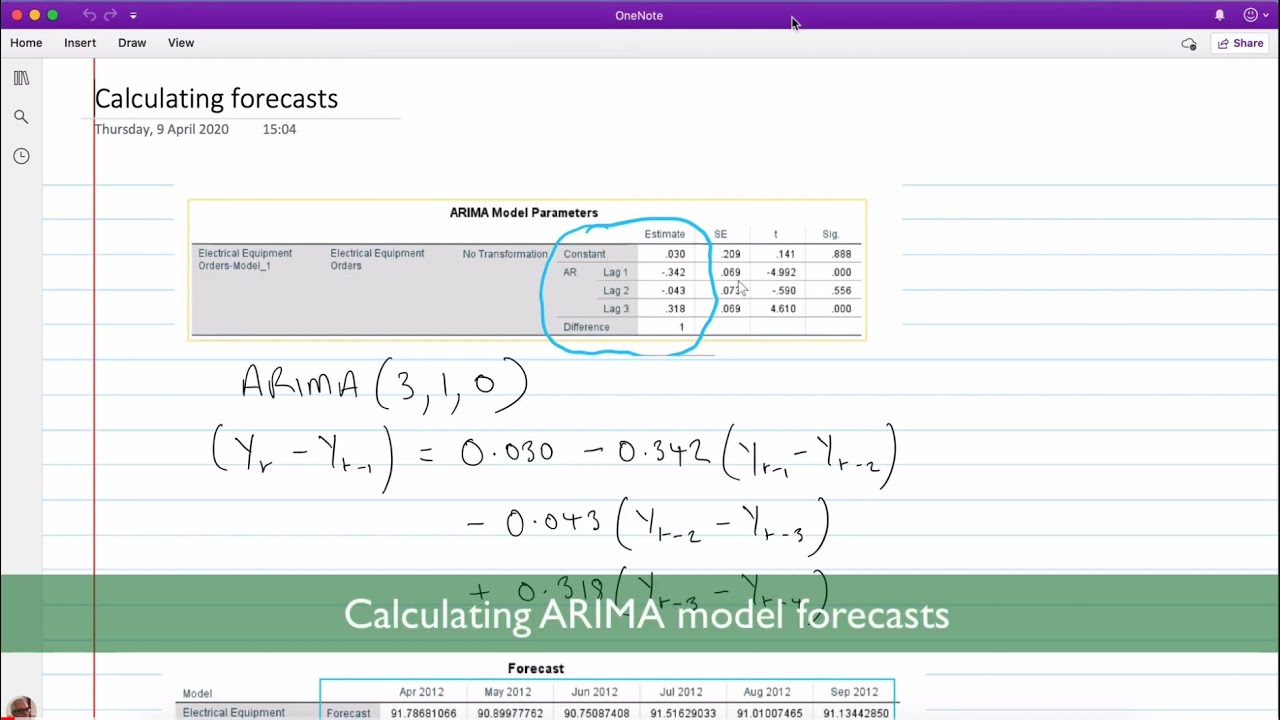
What should i use in my case, arima model or linear regression, and what does arima model has to offer than regression does not for it to compensate for being more complicated.
When to use arima. In this tutorial, we will aim to produce reliable forecasts of time series. If a time series has a trend or seasonality component, it must be made stationary before we can use arima to forecast. Exploratory data analysis and transform data into stationary data.
What is sarima? In that case, it is generally considered better to use a sarima (seasonal arima) model than to increase the order of the ar or ma parts of the model. Arima is a model which is used for predicting future trends on a time series data.
Examples of time series data include s&p 500 index, disease rates, mortality rates, blood pressure tracking, global temperatures. It is model that form of regression analysis. Using arima model, you can forecast a time series using the series past values.
Autoregressive integrated moving average, or arima, is a forecasting method for univariate time series data. However, it is necessary to make sure that the time series is stationary over the historical data of observation overtime period. Arima models are, in theory, the most general class of models for forecasting a time series which can be made to be “stationary” by differencing (if necessary), perhaps in conjunction with nonlinear transformations such as logging or deflating (if necessary).
In this simple guide we break down what an arima model is, how it works, where it's used, and the limitations to keep in mind when building and using them. This equation is generated through three separate parts which can be described as: Model that shows a changing variable that.
An autoregressive integrated moving average, or arima, is a statistical analysis model that uses time series data to either better understand the data set or to predict future trends. About the arima model the parameters used and assumptions made by the model. The arima model aims to explain data by using time series data on its past values and uses linear regression to make predictions.
In this article, i will discuss step 2, using ar,. Sarima similarly uses past values but. Arima models are a powerful tool for analyzing time series data to understand past processes as well as for forecasting future values of a time series.
In this post, we build an optimal arima model from scratch and extend it to seasonal arima (sarima) and sarimax models. Arima models combine autoregressive models and moving average models to give a forecaster a highly parameterizable tool that can be used with a wide variety of time series data. Ar, ma, arma, and arima models are used to forecast the observation at (t+1) based on the historical data of previous time spots recorded for the same observation.
Step 1 — check stationarity: The model is used to understand past data or predict future data in a series. Here is a screenshot of my arima forecast, and.
Arima models are designed to be used in situations where you have one or more univariate time series that you want to forecast independently. Arima takes into account the past values (autoregressive, moving average) and predicts future values based on that. It is a class of statistical algorithms that captures the standard temporal dependencies that is unique to a time series data.