Wonderful Info About Why Do We Use Time Series Forecasting Polar Curve Tangent Line
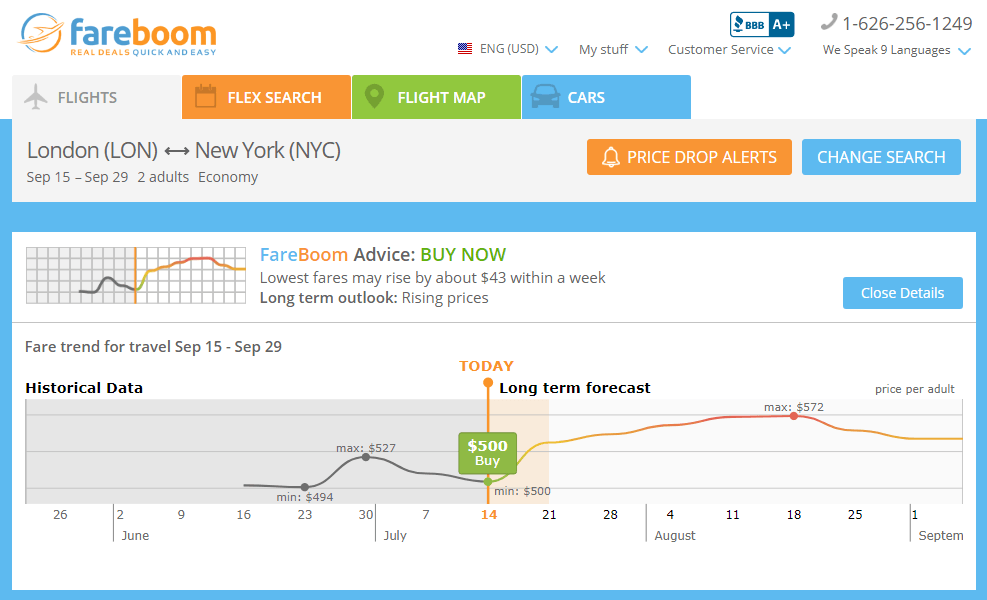
Time series forecasting is a technique in machine learning, which analyzes data and the sequence of time to predict future events.
Why do we use time series forecasting. More than a single number, forecasts are about capturing overall uncertainty by utilizing all. The important components to consider in time series data. In principle, yes, you can see pretty much everything in the original plot, but teasing things apart makes your life easier sometimes.
Time series analysis certainly represents one of the most widespread topics in the field of data science and machine learning: Nate cohn chief political analyst. However, there are other aspects that come into play when dealing with time series.
Time series forecasting occurs when you make scientific predictions based on historical time stamped data. It can show likely changes in the data, like seasonality or cyclic behavior, which provides a better understanding of data variables and helps forecast better. The three types of forecasting.
Exponential smoothing is a forecasting method for univariate time series data. Time series forecasting refers to the practice of examining data that changes over time, then using a statistical model to predict future patterns and trends. Time series forecasting involves analyzing data that evolves over some period of time and then utilizing statistical models to make predictions about future patterns and trends.
After reading this post, you will know: In statistical terms, time series forecasting is the process of analyzing the time series data using statistics and modeling to make predictions and informed strategic decisions. Is the target variable autocorrelated?
What is time series analysis used for? Examples of time series to make your understanding concrete. On 4 july, we have a chance to turn the page and start to rebuild with labour. 22:15:50 analysis:
In this post, you will discover time series forecasting. When forecasting or predicting the future, most time series models assume that each point is. In this post, i will introduce different characteristics of time series and how we can model them to obtain accurate (as much as possible) forecasts.
By establishing expected statistical thresholds and patterns, time series methods can. Without further ado, let’s get started! Time series forecasting is a technique for predicting future events by analyzing past trends, based on the assumption that future trends will hold similar to historical trends.
The most used time series forecasting methods (statistical and machine learning). For forecasting we absolutely need to find the constant (time invariant) component in the series, otherwise it's impossible to forecast by definition. Forms of exponential smoothing extend the analysis to model data with trends and seasonal components.
Time series decomposition helps us disentangle the time series into components that may be easier to understand and, yes, to forecast. Time series analysis can be useful to see how a given asset, security, or economic variable changes over time. Time series forecasting is part of predictive analytics.