Inspirating Tips About Why Exponential Smoothing Is Not Recommended As A Forecasting Method Line Of Symmetry Curve
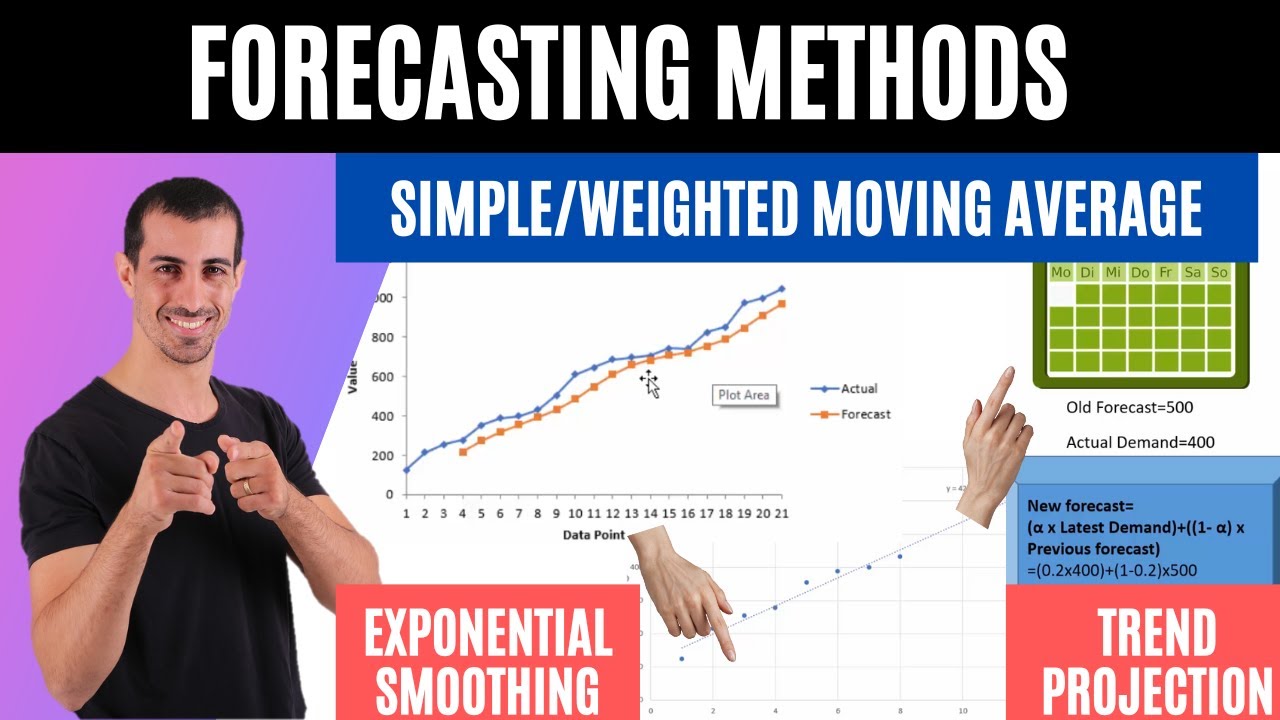
Exponential smoothing is preferred because recent data will have more weight, which consequently aids in giving a more realistic situation at hand and reduces lag.
Why exponential smoothing is not recommended as a forecasting method. Exponential smoothing is a time series forecasting method for univariate data that can be extended to support data with a systematic trend or seasonal component. Single exponential smoothing smoothes the data when no trend or seasonal components are present. It assumes that future patterns will be similar to recent past data and focuses on learning the average demand level over time.
It needs a single parameter called alpha (a), also known as the smoothing factor. Y ^ t = α ( y t + ∑ i = 1 r ( 1 − α) i y t − i), where y ^ t is the forecasted value of the series at time t and α is the smoothing constant. Exponential smoothing is a time series forecasting method that uses an exponentially weighted average of past observations to predict future values.
Exponential smoothing is a family of methods that vary by their trend and seasonal components. Simple exponential smoothing uses a weighted moving average with weights that decrease exponentially. Exponential smoothing is a popular time series forecasting method known for its simplicity and accuracy in predicting future trends based on historical data.
Photo by ocean ng on unsplash. (there is a rise in the last few years, which might suggest a trend. Client asks exponential, but uses different termonology.
This method assigns more weight to recent observations and less to older observations, allowing the forecast to adapt to changing trends in the data. These algorithms, called exponential smoothers, have been in wide use for many decades. Results of this paper suggest that for a restaurant manager with limited resources, an exponential smoothing model could usually give a very satisfactory forecast of sales.
Note that r < t, but r does not have to equal t − 1. There can be no trend (n), an additive (a) linear trend from the forecast horizon, or a damped additive (a d) trend leveling off from the forecast horizon. The quality of these decisions can only be as good as the quality of the forecast upon which they are based.
It is one of the most important tasks, as many other organizational decisions are based on a forecast of the future. Exponential smoothing is a statistical technique that uses past observations of a time series to forecast its future values. We can apply each of these components either additively or multiplicatively.
Key components of exponential smoothing forecast This method is suitable for forecasting data with no clear trend or seasonal pattern. Exponential smoothing is a forecasting method using weighted averages of past data.
Although this demand forecasting method is a little more complicated, it provides some distinct advantages over the two simpler methods. Exponential smoothing methods combine error, trend, and season. This can be a trend that is increasing or decreasing at a constant rate.
The simple exponential smoothing is not able to predict what would be observed at + based on the raw data up to , while the double exponential smoothing and triple exponential smoothing can be used for the prediction due to the presence of as the sequence of best estimates of the linear trend. As i am not in to econometrics so much, first i have to distingquish all termonology client uses. Holt’s method is an extension of a simple exponential smoothing model that allows you to model data that has a constant trend.